Xin Yao
Southern University of Science and Technology (SUSTech), Shenzhen, China
University of Birmingham, UK
Xin Yao will give this talk on the occasion of receiving the 2020 IEEE Frank Rosenblatt Award.
When Everything Else Fails, Try Co-evolution
Co-evolution has been an old research topic in evolutionary computation, but never very popular. It seems to be a last resort, instead of the first choice, when tackling difficult problems. For example, in early days of co-evolutionary learning of game-playing strategies, e.g., strategies for playing iterated prisoner's dilemma games, we faced a hard learning problem where there is no training data and zero knowledge about the game-playing. Yet we have to learn to play the game. Somewhat unexpectedly, co-evolution rose to the challenge and evolved expert level strategies from a set of random strategies with no training data and zero knowledge.Then research on co-evolution got quiet until new challenges come, e.g., the challenge of scalability for evolutionary optimisation. Evolutionary algorithms (EAs) have been successful in solving optimisation problems in various domains, from car engine design to agricultural land use optimisation. However, the number of real-valued decision variables (i.e., problem dimension) that could be handled by EAs is relatively small, up to a couple of hundreds in most cases. Large-scale global optimisation (LSGO) has been a real challenge. Although parallel and distributed computing could help to alleviate the situation a little, the amount of hardware needed can never catch up the growth of optimisation problem. Seemingly as a last resort, the good old co-evolution was brought back to the front stage and used to tackle LSGO. It has now become one of the most effective approaches to LSGO in the literature. Co-evolution has grown out of laboratories. Similar ideas to those used in tackling LSGO have been used in design optimisation in concurrent engineering. Of course, not only can co-evolution be used for numerical global optimisation, it can also be used for combinatorial optimisation, albeit as a last resort again. It is the last resort because the first reaction people would have when hearing combinatorial optimisation would be classical computer algorithms and mathematical programming methods. Only when they fail would one start exploring heuristics and then meta-heuristics. When they also fail, one might think of co-evolution, as a special technique for meta-heuristics. In this talk, we would like to argue, through the previous examples, that co-evolution should probably be treated as a problem-solving approach, rather than just some algorithms or techniques. It encourages us to approach hard problems differently. Finally, it might be worth mentioning that co-evolution theories are also making progresses although at a rather slow pace.
Biography:
Xin Yao obtained his Ph.D. in 1990 from the University of Science and Technology of China (USTC) in Hefei, MSc in 1985 from North China Institute of Computing Technologies in Beijing and BSc in 1982 from USTC.
He is a Chair Professor of Computer Science at the Southern University of Science and Technology (SUSTech), Shenzhen, China, and a part-time Professor of Computer Science at the University of Birmingham, UK. He is an IEEE Fellow and was a Distinguished Lecturer of the IEEE Computational Intelligence Society (CIS). He was the President (2014-15) of IEEE CIS and the Editor-in-Chief (2003-08) of IEEE Transactions on Evolutionary Computation. His major research interests include evolutionary computation, ensemble learning, and their applications to software engineering. His research work won the 2001 IEEE Donald G. Fink Prize Paper Award; 2010, 2016 and 2017 IEEE Transactions on Evolutionary Computation Outstanding Paper Awards; 2011 IEEE Transactions on Neural Networks Outstanding Paper Award; and many other best paper awards at conferences. He received a prestigious Royal Society Wolfson Research Merit Award in 2012, the IEEE CIS Evolutionary Computation Pioneer Award in 2013 and the 2020 IEEE Frank Rosenblatt Award.
Marco Dorigo
Université Libre de Bruxelles, Belgium
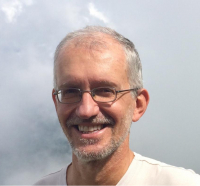
Towards robust and secure robot swarms
Before robot swarms can be deployed in the real world, we need to make them robust to undesired behavior due to the presence of malfunctioning or malicious robots. In the talk, I will first discuss the concept of mergeable nervous system and present experimental results that show how it can be used to design and implement swarms of robots that are easier to control and that can self-repair. I will then discuss how the integration of block chain technology can protect robot swarms from the nefarious effects of the bad behavior of individual robots in the swarm and present results obtained in simulation and with a swarm of pi-puck robots.
Biography:
Marco Dorigo received the Laurea, Master of Technology, degree in industrial technologies engineering in 1986, and the Ph.D. degree in electronic engineering in 1992 from the Politecnico di Milano, Milan, Italy, and the title of Agrégé l'Enseignement Supérieur, from ULB, in 1995. From 1992 to 1993, he was a Research Fellow at the International Computer Science Institute, Berkeley, CA. In 1993, he was a NATO-CNR Fellow, and from 1994 to 1996, a Marie Curie Fellow. Since 1996, he has been a tenured Researcher of the FNRS, the Belgian National Funds for Scientific Research, and co-director of IRIDIA, the artificial intelligence laboratory of the ULB. He is the inventor of the ant colony optimization metaheuristic. His current research interests include swarm intelligence, swarm robotics, and metaheuristics for discrete optimization. He is the Editor-in-Chief of Swarm Intelligence, and an Associate Editor or member of the Editorial Boards of many journals on computational intelligence and adaptive systems. Dr. Dorigo is a Fellow of the AAAI, EurAI, and IEEE. He was awarded the Italian Prize for Artificial Intelligence in 1996, the Marie Curie Excellence Award in 2003, the Dr. A. De Leeuw-Damry-Bourlart award in applied sciences in 2005, the Cajastur International Prize for Soft Computing in 2007, an ERC Advanced Grant in 2010, the IEEE Frank Rosenblatt Award in 2015, and the IEEE Evolutionary Computation Pioneer Award, awarded in 2016.
Zbigniew Michalewicz
Complexica, Australia
Zbigniew Michalewicz will give this talk on the occasion of receiving the 2019 IEEE CIS Evolutionary Computation Pioneer Award.
AI-based business applications and the EC research
The main focus of the talk would be on AI-based business applications for transforming data into decisions, based on variety of large-scale projects executed for three companies (NuTech Solutions, SolveIT Software, and Complexica) over the last 20 years. From the perspective of such applications, several observations would be presented that relate to the current research in the EC community. The topics would include continuous versus discrete optimization, optimization of multi-component problems, single-objectivevs. multi-objective problems, the nature of optimization in dynamic environments, strategic vs. tactical optimization, synchronization between different time horizons, and explanatory features of the optimizers.
Biography:
Zbigniew Michalewicz is the Chief Scientist of Complexica, an Artificial Intelligence software company that helps large organisations sell more products and services, at a higher margin, through the use of automated analytics. He is also Emeritus Professor at the School of Computer Science, University of Adelaide and holds Professor positions at the Institute of Computer Science, Polish Academy of Sciences, at the Polish-Japanese Academy of Information Technology. He has published over 300 articles and 15 books; these include a monograph Genetic Algorithms + Data Structures = Evolution Programs (3 editions, a few translations), Adaptive Business Intelligence, the scientific bestseller How to Solve It: Modern Heuristics (2 editions, a few translations). Other books include Handbook of Evolutionary Computation, Winning Credibility: A guide for building a business from rags to riches, Puzzle-Based Learning: An Introduction to critical thinking, mathematics, and problem-solving, A Guide to Teaching Puzzle-based Learning, and the very recent (2021) The Rise of Artificial Intelligence: Real-world applications for revenue and margin growth.
Zbigniew Michalewicz has over 35 years of academic and industry experiences. He was the co-Founder and Chief Scientist of NuTech Solutions, which was acquired by Netezza and subsequently by IBM, and the co-Founder and Chief Scientist of SolveIT Software, which was acquired by Schneider Electric after becoming the 3rd fastest growing company in Australia. During his time in the corporate world, Professor Michalewicz led numerous large-scale predictive analytics and optimisation projects for major corporations, including Ford Motor Company, BHP Billiton, U.S. Department of Defence, and Bank of America. Professor Michalewicz also served as the Chairman of the Technical Committee on Evolutionary Computation, and later as the Executive Vice President of IEEE Neural Network Council. In December 2013 he was awarded (by the President of Poland, Mr. Bronislaw Komorowski) the Order of the Rebirth of Polish Polonia Restituta.
Carlos Artemio Coello Coello
CINVSTAV-IPN, Mexico City, Mexico
Carlos Artemio Coello Coello will give this talk on the occasion of receiving the 2021 IEEE CIS Evolutionary Computation Pioneer Award.
Lessons Learned after 25 Years Designing Multi-Objective Evolutionary Algorithms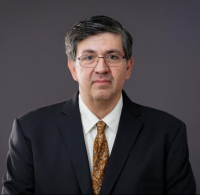
In this talk, I will describe my origins as a researcher working in a field which is known as "evolutionary multi-objective optimization" (EMOO), 25 years ago. Than, I'll describe the inception of my research group at Cinvestav (in Mexico City, Mexico) in 2001, including some of the issues (good and bad) that researchers had to face at that time in Mexico. I will also mention some of the main differences between the way in which research on EMOO was conducted 25 years ago with respect to current research practices including, among other things, topics and methodological issues. In the last part of the talk, I'll briefly mention some of the pioneering contributions developed at my research group, but not without mentioning some of our biggest failures, too. The discussion will include some of the research topics in which I am currently interested. I'll finalize the talk with my personal (and a bit critical) view of the way in which research on EMOO is being conducted today, including some advice for young researchers working in this exciting field.
Biography:
Carlos Artemio Coello Coello received a PhD in Computer Science from Tulane University (USA) in 1996. His research has mainly focused on the design of new multi-objective optimization algorithms based on bio-inspired metaheuristics (e.g., evolutionary algorithms), which is an area in which he has made pioneering contributions. He currently has more than 500 publications, including more than 160 journal papers and 55 book chapters. He has published a monographic book and has edited 3 more books with publishers such as World Scientific and Springer. He has supervised 22 PhD theses (including 3 in Argentina) and 48 Masters thesis (including one in France). Several of the PhD theses that he has supervised, have received awards in national competitions. He has also received (with his students) several “best paper awards” at different international conferences. He is also the only Latin American who has been awarded (twice) the “outstanding paper award” of the IEEE Transactions on Evolutionary Computation. His publications currently report 54,935 citations in Google Scholar. According to Scopus, Dr. Coello has 21,712 citations, excluding self-citations and citations from all his co-authors. His h-index is 94, according to Google Scholar, 65 according to Scopus and 60 according to the ISI Web of Science. In the Shanghai Ranking’s Global Ranking of Academic Subjects 2016 developed by Elsevier, he appears as one of the 300 most highly cited scientists in the world in “Computer Science”, occupying the first place in Mexico.
He has received several awards, including the National Research Award (in 2007) from the Mexican Academy of Science (in the area of exact sciences), the 2009 Medal to the Scientific Merit from Mexico City's congress, the Ciudad Capital: Heberto Castillo 2011 Award for scientists under the age of 45, in Basic Science, the 2012 Scopus Award(Mexico's edition) for being the most highly cited scientist in engineeringin the 5 years previous to the award and the 2012 National Medal of Science in Physics, Mathematics and Natural Sciences from Mexico's presidency (this is the most important award that a scientist can receive in Mexico). He also received the Luis Elizondo Award from the Tecnológico de Monterrey in 2019. Additionally, he is the recipient of the 2013 IEEE Kiyo Tomiyasu Award, "for pioneering contributions to single- and multiobjective optimization techniques using bioinspired metaheuristics", of the 2016 The World Academy of Sciences (TWAS) Awardin “Engineering Sciences”, and of the 2021 IEEE Computational Intelligence Society Evolutionary Computation Pioneer Award. Since January 2011, he is an IEEE Fellow. He is currently the Editor-in-Chief of the IEEE Transactions on Evolutionary Computation.
He is Full Professor with distinction (Investigador Cinvestav 3F) at the Computer Science Department of CINVESTAV-IPN in Mexico City, Mexico.
Roman Słowiński
Poznań University of Technology, Poland
Systems Research Institute Polish Academy of Sciences, Warsaw, Poland
Evolutionary Multiobjective Optimization with Humans in the Loop
Evolutionary Multiobjective Optimization (EMO) algorithms have shown remarkable effectiveness in approximating in the objective space the whole set of Pareto-optimal solutions (called Paretofront) to complex multiobjective optimization problems. However, visualizing such a Pareto front is problematic in the case of more than three objectives, hampering the Decision Maker (DM) to choose the solution that ensures the best compromise between the objectives. For this reason, interactive EMO methods have been proposed, involving the DM in the evolutionary search process. In the interactive EMO, preference elicitation phases alternate with optimization phases. In the preference elicitation phase, the DM is asked to express her preferences on a small subset of solutions from the current population. This preference information is converted to DM’s preference model that makes a pressure on the recombination process, directing the evolutionary optimization towards the part of the Pareto front containing the best compromise solution. For interactive EMO with human DM in the loop, it is important the DM understands the impact of her preference information on the direction of optimization convergence. This affects the psychological convergence of the procedure because the DM should become convinced that her preferences built during subsequent iterations are properly represented and used in the optimization phases. We will review several recently proposed interactive EMO methods, focusing on aspects of faithful, efficient, and explainable representations of DM’s preferences.
Biography:
Roman Słowiński is a Professor and Founding Chair of the Laboratory of Intelligent Decision Support Systems at Poznań University of Technology, and a Professor in the Systems Research Institute of the Polish Academy of Sciences. As an ordinary member of the Polish Academy of Sciences he is its Vice President, elected for the term 2019-2022. He is a member of Academia Europaea and Fellow of IEEE, IRSS, INFORMS and IFIP. In his research, he combines Operational Research and Artificial Intelligence for Decision Aiding. Recipient of the EURO Gold Medal by the European Association of Operational Research Societies (1991), and Doctor HC of Polytechnic Faculty of Mons (Belgium, 2000), University Paris Dauphine (France, 2001), and Technical University of Crete (Greece, 2008). In 2005 he received the Annual Prize of the Foundation for Polish Science - the highest scientific honor awarded in Poland, and in 2020 - the Scientific Award of the Prime Minister of Poland. Since 1999, he is the principal editor of the European Journal of Operational Research (Elsevier), a premier journal in Operational Research.
Risto Miikkulainen
University of Texas at Austin, USA
Cognizant AI Labs, USA,
Risto Miikkulainen will give this talk on the occasion of receiving the 2020 IEEE CIS Evolutionary Computation Pioneer Award.
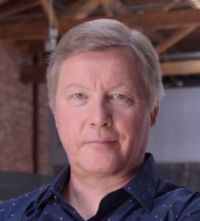
Neuroevolution: A Synergy of Evolution and Learning
Neural network weights and topologies were originally evolved in order to solve tasks where gradients are not available. Recently, it has also become a useful technique for metalearning architectures of deep learning networks. However, neuroevolution is most powerful when it utilizes synergies of evolution and learning. In this talk I review four examples of such synergies: evolving loss functions, co-adapting learning and evolution, evolving activation functions, and evolving decision-making based on surrogate learning. I will demonstrate these synergies in image recognition, game playing, and pandemic policy optimization, and point out opportunities for future work.
Biography:
Risto Miikkulainen is a Professor of Computer Science at the University of Texas at Austin and Associate VP of Evolutionary AI at Cognizant. He received an M.S. in Engineering from Helsinki University of Technology (now Aalto University) in 1986, and a Ph.D. in Computer Science from UCLA in 1990. His current research focuses on methods and applications of neuroevolution, as well as neural network models of natural language processing and vision; he is an author of over 450 articles in these research areas. At Cognizant, and previously as CTO of Sentient Technologies, he is scaling up these approaches to real-world problems. Risto is an IEEE Fellow; his work on neuroevolution has recently been recognized with the IEEE CIS Evolutionary Computation Pioneer Award, the Gabor Award of the International Neural Network Society and Outstanding Paper of the Decade Award of the International Society for Artificial Life.